Why Is DeepSeek So Cheap? The AI Cost Efficiency That's Reshaping the Industry
The meteoric rise of DeepSeek has stunned the AI industry, not just for its technical prowess but for its ability to deliver cutting-edge AI models at a fraction of the cost of Western competitors. With its flagship model, DeepSeek-R1, reportedly trained for just $5.6-6 million-a tenth of Meta's $60 million spend on LLaMA 3 and significantly less than OpenAI's budget for GPT-4-DeepSeek has redefined AI cost efficiency.
Why is DeepSeek so cheap? This article explores the technological, strategic, and geopolitical factors that allow DeepSeek to reduce AI development costs while maintaining competitive performance.
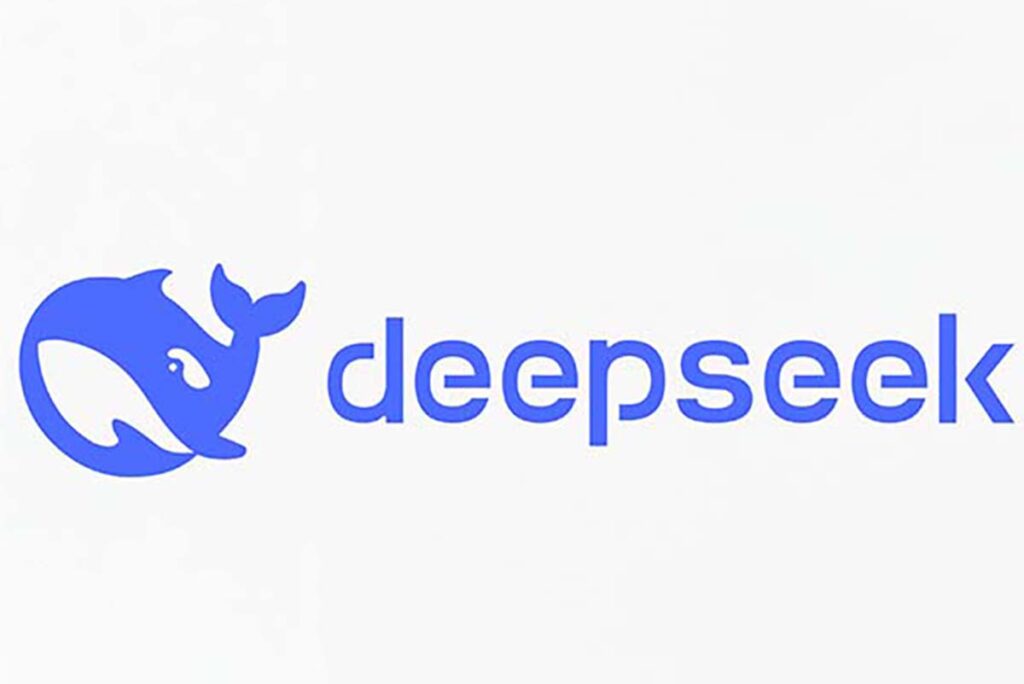
Part 1: Innovative Architecture Drives Cost Savings
At the core of DeepSeek's affordability is its software-first approach, which maximizes computational efficiency through architectural innovations.
Mixture of Experts (MoE) Model
- Unlike traditional models that activate all parameters for every task, DeepSeek's MoE framework divides the model into specialized “experts,” activating only the relevant ones.
- DeepSeek-V3 uses only 37 billion active parameters out of 671 billion total, reducing computational overhead by 80%.
8-Bit Precision Training
- By adopting FP8 (8-bit floating-point) precision instead of higher formats like BF16 or FP32, DeepSeek reduces memory usage by up to 50% while maintaining accuracy.
- This allows training larger models on fewer GPUs, cutting hardware costs significantly.
Multi-Head Latent Attention (MLA)
- MLA compresses memory usage by focusing on critical contextual data, similar to remembering the “essence” of a book rather than every word.
- Combined with sparse activation, it minimizes redundant calculations, improving model efficiency.
These innovations allow DeepSeek to match the performance of models like GPT-4 and Claude 3.5 while using far fewer resources.
Part 2: Hardware Constraints as a Catalyst for Efficiency
Why is DeepSeek so cheap despite hardware limitations? The answer lies in U.S. export controls, which forced DeepSeek to optimize with restricted GPUs like the NVIDIA H800, a downgraded version of the H100 designed for the Chinese market.
Optimized GPU Utilization
- The H800's reduced NVLink bandwidth (400 GB/s vs. H100's 900 GB/s) initially slowed inter-GPU communication.
- DeepSeek bypassed NVIDIA's CUDA framework, using low-level PTX programming to directly control GPU cores, compensating for bandwidth gaps and achieving 90%+ GPU utilization.
Custom Communication Protocols
- DeepSeek developed proprietary algorithms, such as the HAI-LLM framework, to optimize task distribution, eliminating idle GPU time.
Scaling with Smaller Clusters
- While Meta trained LLaMA 3 on 16,000 GPUs, DeepSeek-V3 required only 2,048 H800s, reducing infrastructure costs and energy consumption.
By turning hardware constraints into a competitive edge, DeepSeek demonstrated that raw compute power isn't the only path to AI supremacy.
Part 3: Cost-Efficient Training Practices
Beyond AI model architecture, DeepSeek's training methodology is also optimized for cost efficiency.
Synthetic Data and Knowledge Distillation
- DeepSeek reduces data acquisition costs by relying on synthetic data, generated by smaller models like DeepSeek-R1 Lite, instead of expensive human-annotated datasets.
Reinforcement Learning Optimization
- DeepSeek R1 uses reinforcement learning to minimize trial-and-error cycles during training, reducing wasted computation.
Partial 8-Bit Training
- Instead of quantizing the entire model, DeepSeek applies selective 8-bit quantization to specific weights and optimizer states, doubling memory efficiency while maintaining accuracy.
These practices allow DeepSeek to train AI models like V3 in under two months, compared to Meta's multi-year LLaMA 3 development cycle.
Part 4: Open-Source Strategy and Ecosystem Leverage
One of the biggest reasons why DeepSeek is so cheap is its open-source AI model strategy.
Community-Driven Innovation
- DeepSeek R1 and V3 were released under the MIT license, encouraging global contributions that accelerate improvements without increasing R&D costs.
API Pricing Disruption
- DeepSeek's API pricing is $0.55 per million input tokens, which is 3.7% of OpenAI's $15 per million tokens.
- This attracts startups and independent researchers, expanding DeepSeek's user base without requiring massive marketing expenses.
Distilled AI Models
- DeepSeek offers smaller, specialized AI models like DeepSeek-R1 Lite, enabling cost-conscious businesses to deploy AI on minimal GPU resources.
This mirrors the success of Linux, proving that open-source AI models can compete with proprietary AI giants.
Part 5: Geopolitical and Market Factors
The U.S.-China AI race has unexpectedly contributed to DeepSeek's cost efficiency.
Export Restrictions as Innovation Fuel
- DeepSeek was denied access to NVIDIA's H100 GPUs, so it optimized for H800s, proving that software ingenuity can offset hardware gaps.
Lower Labor and R&D Costs
- With a team of engineers from top Chinese universities, DeepSeek maintains lower R&D costs compared to Silicon Valley AI startups.
Domestic Market Focus
- DeepSeek first targeted the Asian AI market, refining its cost-effective AI models before expanding globally.
These factors further enhance DeepSeek's ability to offer AI at a lower cost.
Part 6: Challenges and Skepticism
Despite its low-cost AI revolution, DeepSeek faces several challenges.
Hidden Costs
- Some analysts argue that DeepSeek's $6 million figure excludes pre-training experiments, data collection, and operational expenses.
- Real costs may exceed $500 million when including infrastructure investments.
Scalability Concerns
- DeepSeek's training efficiency is optimized for smaller clusters, but as models grow, scaling might become more difficult.
Geopolitical Risks
- Western AI markets may be hesitant to adopt Chinese AI models due to trust issues and regulatory concerns.
Conclusion
Why is DeepSeek so cheap? The answer lies in efficiency, innovation, and geopolitical strategy. By prioritizing cost-effective AI model training, optimizing hardware use, and leveraging open-source AI models, DeepSeek has rewritten AI's economic playbook.
Its affordability forces competitors like NVIDIA and OpenAI to rethink AI development costs, proving that brute-force computing power is no longer the only path forward.
As DeepSeek's founder Liang Wenfeng put it, We calculated costs and set prices accordingly. In an era where AI's impact depends on accessibility, DeepSeek's pricing strategy may be as transformative as its technology.
Share this article:
Select the product rating:
Daniel Walker
Editor-in-Chief
My passion lies in bridging the gap between cutting-edge technology and everyday creativity. With years of hands-on experience, I create content that not only informs but inspires our audience to embrace digital tools confidently.
View all ArticlesLeave a Comment
Create your review for HitPaw articles